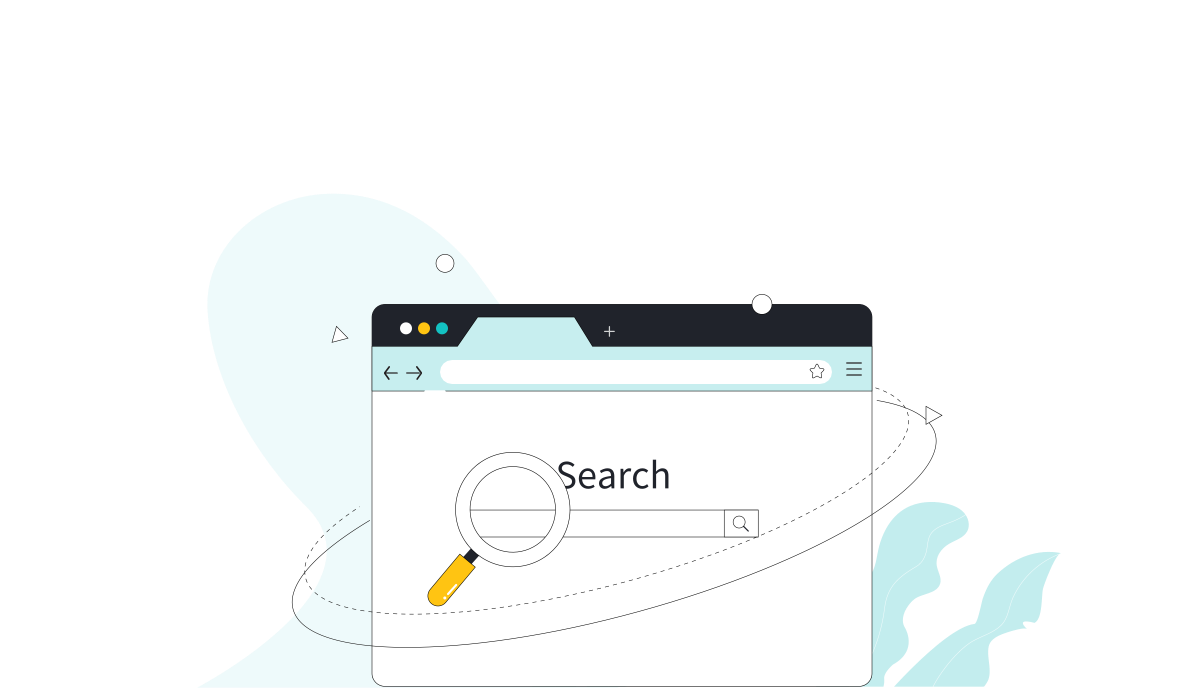
Government open data is vast and spans a wide range of business domains. Users, when searching for specific information within this massive dataset, face limitations such as keyword-matching constraints, low relevance recommendations, slow search speeds, and suboptimal data quality. This results in users spending considerable time and effort filtering and organizing data, hindering the realization of the maximum value of open data.
We pioneered the creation of the "Field Search" model nationwide, breaking down barriers between tables. The core of this approach is rule-based auto-discovery, combining logical rules with machine learning for field-level data mining and correlation analysis. We have established a data quality assurance system that leverages Rock's data quality system capabilities to enhance data quality, ensuring accuracy and completeness.
Poor User Experience. Low relevance between search results and recommendations.
Data Lag. Manual discovery of data quality issues, leading to significant lag.
High Volume of Dirty Data. Numerous quality issues in source data, including null values, format errors, and multiple data entry points.
Open data field-level analysis enables efficient and precise discovery of inter-data relationships, providing more refined data search services and accurate data correlation recommendations.
Automated Discovery of Data Quality Issues. Enhanced data quality through intelligent capabilities like entity resolution, conflict resolution, and data completion, addressing issues of data lag, dirty data, and missing data in a visually intuitive manner.
Since its launch, the overall data quality of the platform has improved by 35%.
The number of data interface calls has increased by 179 million times.
The relevance and quality of search results have significantly improved, leading to a 30% overall increase in user satisfaction.