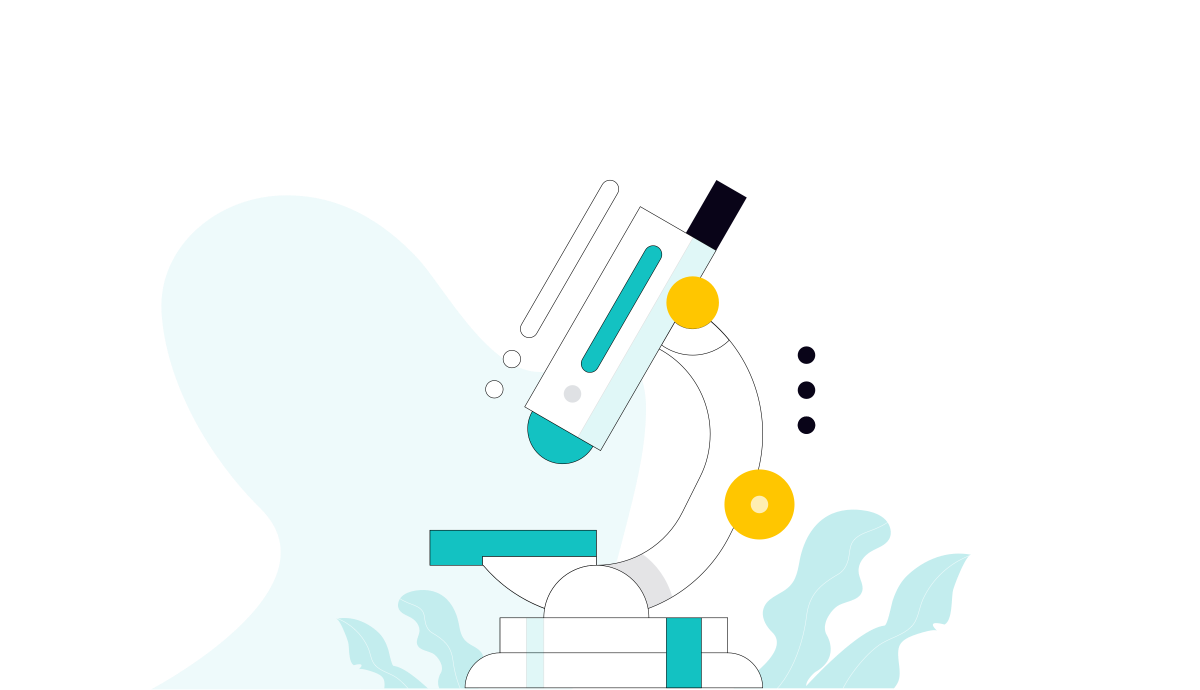
A certain research institute in the field of science primarily focuses on providing information assurance for scientific literature in natural sciences, interdisciplinary sciences, and high-tech fields, along with conducting strategic intelligence research services. Its data is sourced globally from research institutions, higher education establishments, and journals. Data from various origins often contain multiple aliases and abbreviations, leading to issues like inconsistency and redundancy. The institute faces challenges in rapidly identifying and retrieving data that describes the same entity but with different expressions. The current approach involves manual queries for identification, which is inefficient and has a low accuracy rate. Resolving the issue of entity uniqueness in big data quickly and efficiently stands as a major pain point for this institution.
The Rock System employs machine learning models within the data to automatically match and filter based on data features. This enables semantic judgment of entities, automatic discovery of entity uniqueness rules, and the organic integration of similarity algorithms and machine learning models. The system provides a rapid and convenient human-machine interaction, presenting identified entities clearly. This approach reduces manual intervention, significantly enhancing the efficiency of data processing.
Dependency on manually designed rules results in low accuracy.
Disorganized data, making it challenging to manually classify and identify.
Offer rapid and convenient human-machine interaction, presenting identified entities clearly.
Automatically discover the same entities and perform automatic clustering.
Identified and deduplicated 450,000 repeated entries within a dataset of 4.5 million organizations. Automated the filling of missing data with an accuracy rate reaching 95.4%.
Completed operations on a million-level dataset within minutes, showcasing a 100-fold improvement in model computation efficiency.
Accelerated entity retrieval speed by more than 50%.