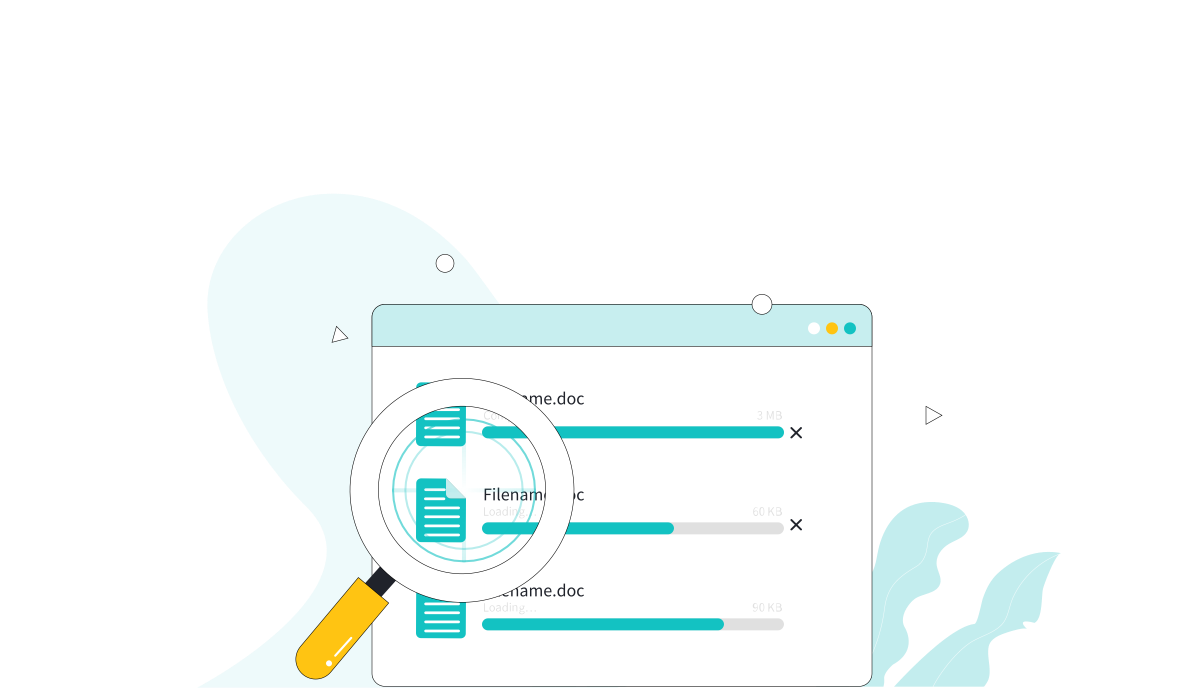
In order to meet regulatory demands, a prominent bank in Shenzhen has created a data anomaly monitoring system. This system is designed to detect fluctuations and abnormal changes in both detailed and summary-type reports. The core functionality of the system relies predominantly on manually crafted rules by business personnel. It performs data error detection on reported data such as the Banking and Insurance Regulatory 1104 report, Banking and Insurance Regulatory EAST submission, and People's Bank of China's credit information for anti-money laundering. However, during the actual implementation process, the complexity arising from the collaboration between various roles, including business processors, business managers, data submitters, data managers, and technical developers, has led to issues in the quality of submitted data. Problems such as "failure to fulfill customer identity recognition obligations as specified" and "inaccurate asset quality classification" have emerged.
Data preprocessing and feature extraction are conducted on the provided indicator and detailed tables. Combining industry knowledge in Banking regulatory reporting and partial data dictionaries, the automatic rule discovery feature of Rock is employed to automatically identify rules for the validation of data integrity and accuracy.
The process heavily relies on manually crafted rules for data error validation.
Identifying and analyzing errors found in regulatory reporting proves to be challenging when done manually.
The utilization of the automatic rule discovery feature significantly enhances the accuracy and coverage of error validation.
Errors discovered through rules can be traced back, enabling a systematic analysis of the root causes.
Automated discovery identified 91 valuable rules, with approximately 10% of these rules being previously unrecognized by manual efforts.
Utilizing the automatically discovered rules for data error validation revealed over 200 instances of erroneous data, triggering alert notifications.