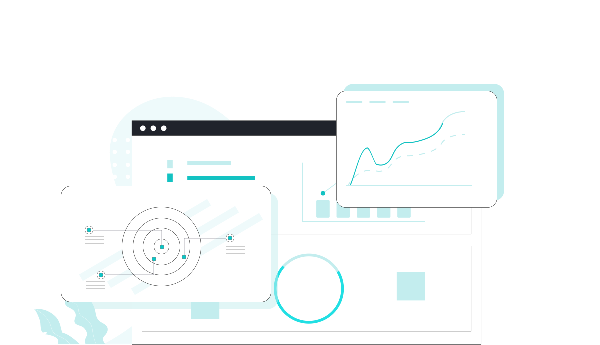
In the transaction flow of corporate clients, there typically exists various risky transaction behaviors such as fraud and money laundering. Currently, the issues of low efficiency, error-proneness, limited timeliness of rule discovery can be found in manual data analysis. As a result, there is a need for automated risk identification based on a combination of basic company information as well as information associated with public security and legal authorities.
By analyzing the data of historical fraud cases, The Wuji intelligent risk identification solution efficiently, flexibly and intelligently identifies the characteristics of fraudulent behavior, automatically constructs the graph structure, analyzes the correlation between entities, automatically mines the fraud risk control rules, and establishes a rule base for real-time monitoring of fraudulent behaviors, enriches the existing rule base, and improves anti-fraud capabilities.
Low accuracy: over-reliance on manually envisioned rules and low accuracy.
Low timeliness:For fraud and other risky customers, transaction behavior is variable and there is a lag in updating risk control rules.
Difficulty in Risk Assessment: The risk rules are not comprehensive, resulting in risk controls that lack judgment about the cause or nature of the risk being created.
Constructing graph data and automatically discovering rules through the network topology relationship of the data to improve the accuracy of the rules.
Supports real-time updating and real-time monitoring of the rule base to improve the efficiency of risk identification.
Comprehensive analysis from multiple sources of data, causal inference through big data analysis, and interpretable results.
31 valuable rules were automatically discovered, with a 30% increase in rule richness.
The test set was labeled by rule results with 82.4% accuracy and 79.8% recall.