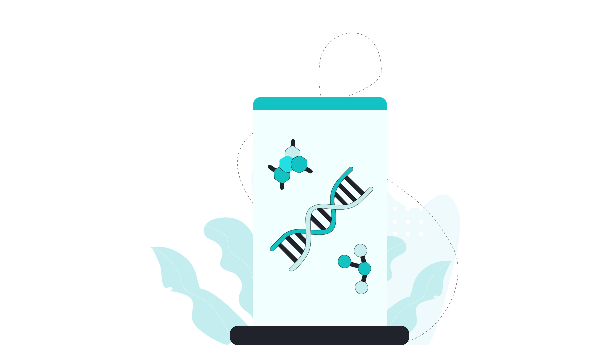
A bio-pharmaceutical laboratory in Shenzhen's Guangming District is engaged in the development of new peptide drugs in key areas such as cancer and metabolism. The traditional experiment-driven R&D model leads to many experiment failures, making the entire drug development process time-consuming and expensive. The laboratory also attempts to use AI models to predict PPI (Protein-Protein Interaction) in the early stages of drug development, hoping to reduce the cost and duration of biological experiments. However, due to the unexplainable results and low accuracy of AI black box models, they are still unable to reduce the failure rate and scope of experiments.
The project utilized the "Qu Bing" system for graph data fusion on the complete protein interaction network of BioGRID. Specifically, ClustalW was used to introduce protein homology information; DAVID provided pathway and domain information related to human proteins; and UniProt supplied subcellular localization information for proteins. On the fused graph data, graph association rules were applied to predict protein interactions with STAT3, ultimately identifying three key protein interaction pairs: STAT3 with CARM1, STAT3 with PARP1, and STAT3 with CTNNB1. Subsequent co-immunoprecipitation (Co-IP) and mass spectrometry experiments verified significant interactions in two of these pairs.
The AI black box model has low accuracy, produces numerous results that are uninterpretable, making it difficult to select candidates for experimentation.
Experimental cycles measured in months, iterative experiments, and a high rate of experiment failure.
Highly precise and explainable predictions lead to high accuracy of experimental results, allowing experts to filter the results based on experience.
Increased success rate in experiments, shortening the overall drug development cycle.
Build richer PPI (Protein-Protein Interaction) network graph data based on open-source data.
n the preliminary recall of protein interactions, compared to black box models (GNN, CNN), the accuracy improved by 43%.
Predicted interactions between three protein groups, and subsequent biological experiments showed significant interactions in two of these pairs.
Predicted that the human protein SYT2 has self-interactions, which was validated in the May 2022 Nature article "Inhibition of calcium-triggered secretion by hydrocarbon-stapled peptides."